A research group has taught AI to magnetically wrangle a high-powered stream of plasma used for fusion research — but wait! Put away your EMPs and screwdrivers, this is definitely a good thing, not a terrifying weapon for use against humanity in the coming robocalypse.
The project is a collaboration between Google’s DeepMind and l’École Polytechnique Fédérale de Lausanne (EPFL) started years ago when AI researchers from the former and fusion researchers from the latter met at a London hackathon. EPFL’s Federico Felici explained the problem his lab was having with plasma maintenance in his tokamak.
Such an everyday complaint! Yet it struck a chord with DeepMind and the two got to work.
Fusion research is conducted in many ways, but all of them involve plasmas formed at incredibly high temperatures — hundreds of millions of degrees. Sounds dangerous, and it is, but a tokamak is one way to keep it under control and allow close observation of the fusion activity happening within. It’s basically a torus or donut through which the superheated plasma travels in a circle, its path carefully constricted by magnetic fields.
To be clear, this isn’t a fusion reactor of the type you hear about giving nearly unlimited clean energy; it doesn’t produce energy, and if it suddenly started, you wouldn’t want to be anywhere nearby. It’s a research tool for testing and observing how these volatile but promising processes can be controlled and used for good.
In particular, the “variable-configuration” tokamak at the Swiss Plasma Center allows not just the containment of a ring of plasma, but for researchers to control its shape and path. By adjusting the magnetic parameters thousands of times per second, the ring can be made wider, thinner, more dense or diffuse, all kinds of factors that might affect its qualities.
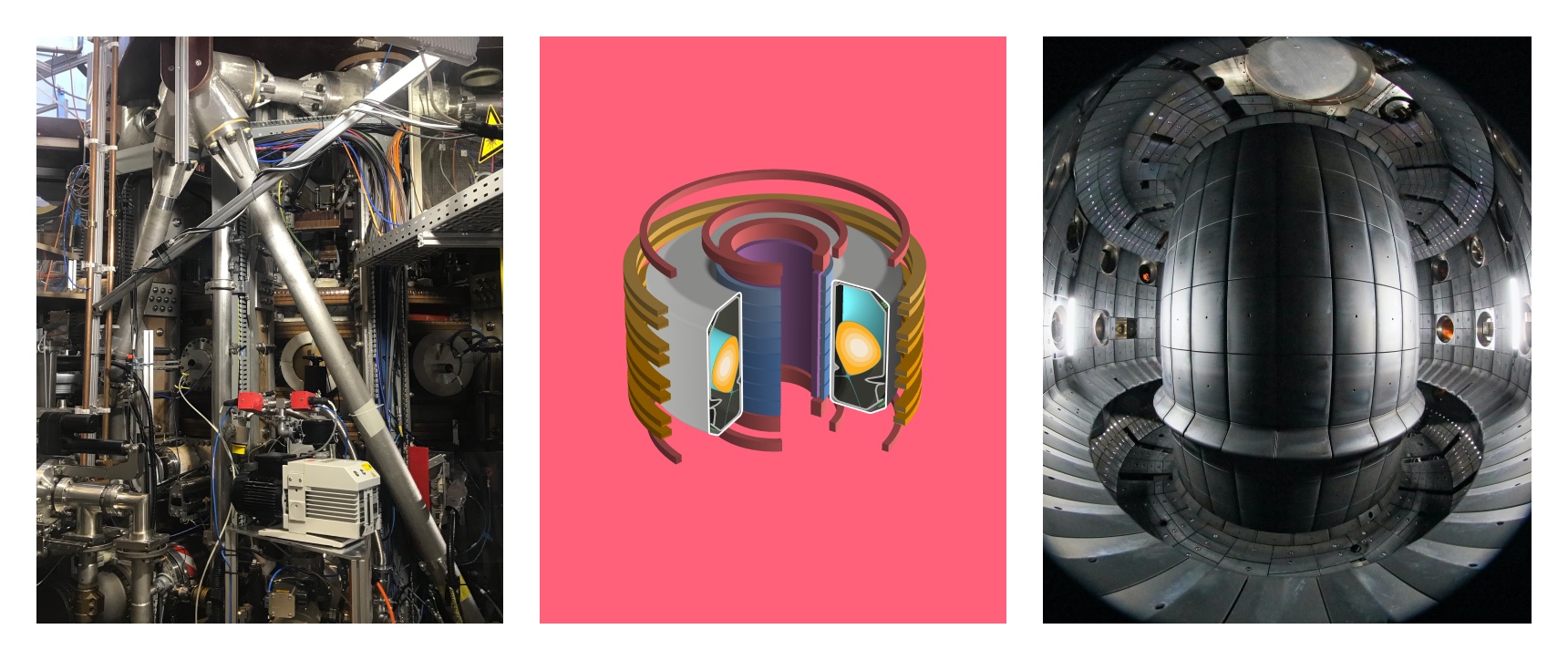
Image Credits: DeepMind & SPC/EPFL
The precise settings for the machine’s magnetic fields must be determined ahead of time, naturally, as the cost of improvising them badly is potentially serious damage. The settings are configured using a powerful simulator of the tokamak and plasma, which the team has been updating for years. But as Felici explained in an EPFL news release: “Lengthy calculations are still needed to determine the right value for each variable in the control system. That’s where our joint research project with DeepMind comes in.”
The teams trained a machine learning system first to predict what plasma pattern a given set of settings would produce, then to work backwards from a desired plasma pattern and identify the settings that would produce it. (Simply stated, not so simply achieved, as is is often the case with AI applications like this.)
According to a paper published today in the journal Nature, the approach was a resounding success:
This architecture meets control objectives specified at a high level, at the same time satisfying physical and operational constraints. This approach has unprecedented flexibility and generality in problem specification and yields a notable reduction in design effort to produce new plasma configurations. We successfully produce and control a diverse set of plasma configurations on the Tokamak à Configuration Variable including elongated, conventional shapes, as well as advanced configurations, such as negative triangularity and ‘snowflake’ configurations.
And here are some examples of different shapes and configurations the model was able to produce:
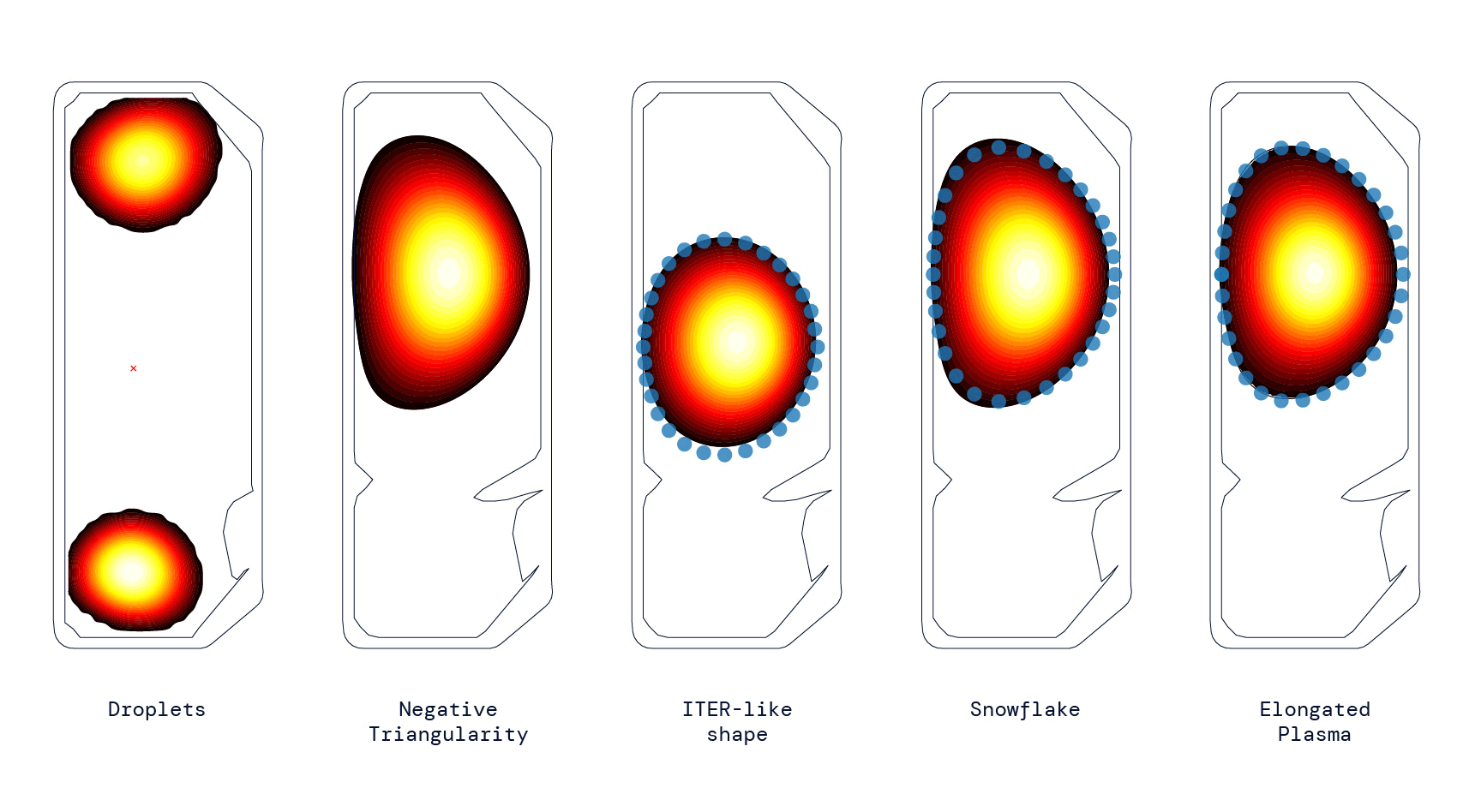
Slice of the tokamak “donut” showing cutaway view of interior and beam. Image Credits: DeepMind & SPC/EPFL
This is important work because experimenting with plasma like this — let alone using it for power — involves lots and lots (think millions) of tiny tweaks and those can’t all be manually configured. If a theory calls for two streams, one 22% larger than the other, it might take weeks or months of work to come up with the theoretical settings to produce that using “traditional” methods (which, to be clear, are already fantastically complex digital simulations). But an AI could come up with a good match in a tiny fraction of that time, either creating the solution right there or giving human auditors a strong starting point to work from.
It also could be important for safety, since no human can improvise settings over a second or two that could contain an anomaly in time. But an AI might be able to change the settings in real time to prevent damage.
DeepMind researcher Martin Riedmiller admitted that it’s “early days,” but of course that can be said for nearly every AI application in science. Machine learning is proving to be a powerful and versatile tool for innumerable disciplines — but like good scientists they are taking every success with a grain of salt and looking forward to the next, more confident result.
from TechCrunch https://ift.tt/tblJLxq
No comments:
Post a Comment
welcome